Authors: Spencer D. Wyld1 and David C. Wyld2
1 Walton College of Business, Department of Finance, University of Arkansas, Fayetteville, AR, USA
2 Department of Management & Business Administration, Southeastern Louisiana University, Hammond, LA, USA
Corresponding Author:
David C. Wyld, DBA
47042 Scott Drive
Hammond, LA 70401
dwyld@selu.edu
985-789-2127
Spencer D. Wyld, M.B.A., is a doctoral candidate in finance in the Walton College of Business at the University of Arkansas with a focus on the financial aspects of energy, infrastructure, and transportation.
David C. Wyld, D.B.A., serves as the Merritt Professor of Management at Southeastern Louisiana University. His research interests involve the intersection of technology, society, sports and business.
College Football’s Bottom-Line Impact: Exploring the Relationship of Football Performance on Athletic Finances for Division I Institutions Today
ABSTRACT
Purpose: This study examines a heretofore unaddressed area in both sport and economics literature, looking at the relationship between on-the-field college football team performance and the financial performance of university athletic operations overall. Methods: The researchers, building upon prior research employing econometrics to sports analysis, utilized data spanning 2005-2018 for 106 Division I college athletic programs to examine how specific aspects of offensive, defensive, and overall team football performance related to four measures of overall athletic department financial performance (donor contributions, corporate sponsorships, ticket sales, and profit). Results: Based on three separate regression analyses, the study found that while institutional and state control variables were important in explaining overall differences in universities’ athletic department performance in terms of donor contributions, corporate sponsorships and ticket sales, some football-specific factors were found to have significance as well. Overall, college athletic departments that had teams which produced exciting football on the field (with scoring and with a lack of turnovers) were found to be better performing off the field financially in contributing to the overall success of their university’s athletic programs. Conclusions: The present research demonstrates for the first time how – and how much – on-field football team performance can play a role in athletic operations overall from a financial standpoint. The researchers go on to analyze directions – and challenges – for future research in this area, especially considering the implications of the COVID-19 pandemic for college sports operations. Applications in Sport: For athletic departments and athletic administrators, the findings in the present research provide new insights on what donors, sponsors, and fans of their football programs value in terms of on-field performance of their respective university’s football team.
Key Words: football, athletics, financial, econometrics, economic analysis, athletic department, NCAA, college sports, university, administration
INTRODUCTION
Football: “The Straw That Stirs the Drink” for College Athletics Financially Today
According to the most recent data from the NCAA (National Collegiate Athletic Association) (28), there are over half a million student athletes in colleges and universities across America today that compete in 24 sanctioned sports. In the case of college athletic departments, the breadth and diversity of sports that universities typically offer their students the opportunity to participate in today is impressive – and often surprising to those outside of the respective institutions – far beyond the spectator sports like football, basketball, and baseball that fill our television screens that draw audiences from across the country today (16).
While the number of specific sports offerings varies greatly based on the size and “athletic intensity” of individual universities, one thing holds true across almost all institutions: From a strategic management perspective (5, 20), football is the key driver of athletic department operations. Both in tangible terms – attendance, donations, sponsorships, and yes, revenue – and in intangible measures, including media coverage, branding and name recognition, along with that most elusive “thing” of all for universities – school “spirit” and identification – football is today, without a doubt, the most important of all college sports in terms of what it means to the university from a financial, operational, and yes, strategic standpoint.
Whether the centrality of football to athletic department operations in universities at present is “right” or not is a topic for another day and certainly, many more journal articles and conferences. However, the fact is that today, football is, as baseball great Reggie Jackson famously put it, “the straw that stirs the drink” (26) in terms of collegiate athletic departments. No less an authority than noted author Malcolm Gladwell (17) has observed that today, “college football is a big business” (n.p.), and as such, it is useful to view football programs in terms of business metrics – including their revenue and yes, their profitability – i.e., the amount of money they generate in excess of their expenses that helps fund, in most cases and to a great extent enable, the remainder of much of college athletics.
Football and Athletics’ Bottom-Line
Just how big and important are college football programs to college and university athletic operations overall today? Each year for the past few years, Forbes magazine has ranked college football programs based on their financial success. The most recent Forbes report (39) demonstrates evidence to show just how important the football program is to the college athletics operations today. Table 1 (Top 10 College Football Programs in Terms of Revenue and Profitability) shows the revenues, expenses and yes, profitability, for the biggest and most successful – in terms of these financial metrics at least – college football teams. These “profits,” which in practical terms are the excess funds generated by the football program, help fund the overall athletic department offerings and operations for institutions.
Table 1: Top 10 College Football Programs in Revenue and Profitability
Rank | Institution | 3 Year Average Revenue (2016-2018) (Millions) | 3 Year Average Expenses (2016-2018) (Millions) | 3 Year Average Gross Profit (2016-2018) (Millions) | 3 Year Average Gross Profit (2016-2018) (Percentage) |
1 | Texas A&M University | $147 | $53 | $94 | 63.95% |
2 | University of Texas | $147 | $55 | $92 | 62.59% |
3 | University of Michigan | $139 | $56 | $83 | 59.71% |
4 | University of Alabama | $134 | $73 | $61 | 45.52% |
5 | Ohio State University | $132 | $57 | $75 | 56.82% |
6 | University of Oklahoma | $129 | $48 | $81 | 62.79% |
7 | University of Georgia | $125 | $51 | $74 | 59.20% |
8 | University of Notre Dame | $120 | $44 | $76 | 63.33% |
9 | University of Florida | $117 | $48 | $69 | 58.97% |
10 | Auburn University | $117 | $52 | $65 | 55.56% |
Average for Top 10 Programs | $131 | $54 | $77 | 58.84% | |
Total for Top 10 Programs | $1,307 | $537 | $770 |
Source Info: “College Football’s Most Valuable Teams: Reigning Champion Clemson Tigers Claw Into Top 25,” by Chris Smith, Forbes, September 12, 2019. Retrieved from https://www.forbes.com/sites/chrissmith/2019/09/12/college-football-most-valuable-clemson-texas-am/#cbcea77a2e7e.
Based on the most recent three years complete data available (from 2016-2018) for the Forbes’ rankings, all of the top 10 ranked football programs generated in excess of $100 million in revenue annually and, with an average profit margin approaching 60% (58.84%), these football programs returned – on average – $77 million dollars a year to their athletic departments to sustain their operations. Collectively, these top 10 football programs alone generated in excess of $1.3 billion dollars in revenue annually in the most recent time period, returning some $770 million to their respective schools’ athletic operations. This demonstrates just how big a business college football is today – and just how important the revenue and profits (i.e., excess funds) are to universities and the operations of their overall athletic programs.
Positioning the Present Study
With the preeminence of football in athletic programs today, not just in terms of attention, but in terms of revenue generation for universities and their overall athletic operations, the present research is geared towards addressing a research gap that has not been addressed to date in either the sports or economics literature. The specific research gap is this: While we can analyze the importance of the relationship between the football program and the overall athletic operation within a university from a purely financial perspective, which is no doubt important today, there has not been a study to date that has employed a widely used method in the fields of sports and economics to use econometrics to examine the intersection of sports performance and economic variables. Thus, this study is unique in that it is an initial attempt to link on-field football team performance (and the derivative end-of-season rewards for such performance) with the financial performance of college athletic programs. As such, it both builds upon prior econometrically based sports research. It is hoped that this study will open-up a whole new way of not just looking at college athletics from a research standpoint, but also helping universities and athletic administrators to better evaluate and manage their football and athletic operations.
The central question in the present research is this: While there are multiple factors that help determine athletic department revenues, is it possible that specific aspects of the performance of a school’s football team could factor into the school’s total athletic department revenue and yes, its profitability, in a predictable manner? And so as to better understand the relationships between on-the-field football team performance and athletic department financial performance, we use econometric analysis to explore the effects of specific aspects of football team performance on four financial measures of athletic department operations:
- Donor contributions,
- Corporate sponsorships,
- Ticket sales, and
- Profit.
Beyond exploring if football team performance causes such effects, we also examine a timing question. This is whether the team’s previous year or current year on-the-field football performance has a stronger effect on financial measures of athletic department operations. The study used data from Sports Reference (for on-the-field football performance) and from the Knight Commission on Intercollegiate Athletics College Athletics Financial Information (CAFI) Database (for university athletic department financial performance).
Review of Related Literature
Econometric analysis of sports performance, antecedents, causation, correlation , and results has a long history of use across many types of sports, both at the team and individual levels. For instance, performance at the Olympic Games has been a particularly lively area of econometric inquiry, with a rich line of inquiry into economic, demographic and social correlations with both national (team) and individual performance. Such research on Olympic performance is exemplified by the works of the likes of Lui and Suen (27), Forrest, Sanz, and Tena (12), and Bernard and Busse (3). as well as econometric analyses of the performance of paralympic teams/athletes (7, 38). Likewise, econometric analysis has been applied to seek determinants of both soccer (football) team performance (see for example the analyses done by Haas, et al. [19] on German football and Pestana-Barros, et al. [32], on Brazilian football). Econometric analyses have even “drilled down” to examine the impact of such global economic and social variables on micro, in-game decision making, including player substitution patterns (9) and referee decisions (35, 36).
Such an econometric approach to analyzing sports performance has been taken wide afield in both the sport and economic literature. Examples of just how far include prior published econometric examinations of economic, social , and demographic variables on sporting outcomes and team/individual results and metrics include prior research on subjects as diverse as:
- Major League Baseball (MLB) (13);
- The National Basketball Association (NBA) (14);
- The National Football League (NFL) (21); and
- Australian Rules Football (AFL) (41).
There has also been econometric modeling used in analyzing in-game performance metrics in the NFL, such as the work of Arkes (1), which examined the importance of offensive elements (running versus passing) in pro football.
College sports, and specifically college football, have seen a fair number of econometric analyses in recent years, with prior studies having looked primarily at college football success (i.e., winning) as a causal variable on the college applications/admissions (33) and on the economics of college towns (2). And while there has been a great deal of research – and discussion – in terms of ranking athletic spending, donations to athletic programs and on the field success in college football (39, 45), there has been a dearth of formal, econometric analyses into college football conducted to date on par with that done on other sports in both the economic and sport literature. Thus, our research seeks to examine college football in a new, novel way and apply econometric analysis to the sport to address this research gap.
METHODS
This section details the research methods employed in the current research. It describes the data incorporated into the study, along with detailing the methodology and the hypotheses of the present research.
Data
In the following sections, the sourcing of the data utilized in the current research is detailed , and the various measures used in the study are defined. The three categories of data are:
- College Athletics Financial Data
- Football Data
- School and State Control Data
College Athletics Financial Data
The source for financial data on college athletics programs is the Knight Commission on Intercollegiate Athletics’ College Athletics Financial Information (CAFI) Database (23). The CAFI database contains a breakdown of the athletic department revenue and expenses of public Division I institutions. As such, the CAFI includes data for both Football Bowl Subdivision (FBS) and Football Championship Subdivision (FCS) schools. Financial data for these universities comes directly from NCAA (National Collegiate Athletic Association) Financial Report Forms that are self-reported annually by each public institution. In total, the database for the present research incorporates financial data spanning the 2005 to 2018 timeframe.
The present study examines only Division I FBS institutions, excluding those universities within the FCS. The decision to remove FCS schools from the database for the current research stems from the vast differences that exist between FBS and FCS institutions. Not only are there profound financial differences to be found between universities operating in the two levels of Division I football (4), but there is also a significant distinction in the overall structure of the football season between FBS and FCS institutions. This is the fact that FBS universities play their regular seasons in hopes of going to one of the many end-of-season bowl games (which becomes an important source of additional revenue for them), while FCS schools compete to be slotted into a national playoff system that affords them to play in a championship-determining tournament, but which does not provide the kind of financial payouts that bowl games provide to institutions.
Thus, after screening the CAFI data to eliminate institutions that did not maintain continuous Division I FBS status, the military academies, and all schools in Pennsylvania (due to a state law precluding financial disclosures,) Figure 1 (Map of the 106 Universities Under Review) shows the locations of all the 106 schools that comprise the final dataset employed in the present research.
Figure 1: Map of the 106 Universities Under Review
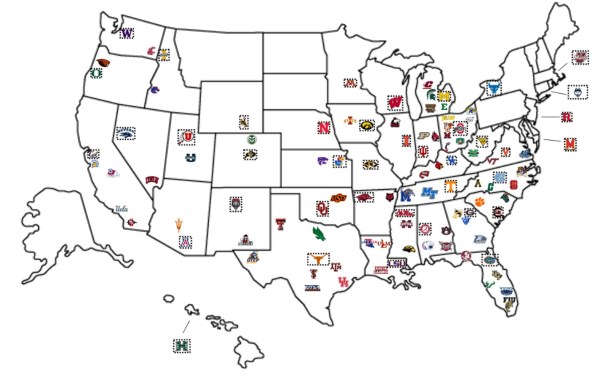
Note:This map features the logos of the university included in this study. Flagship universities are designated with a gridded box around the logo. For full list of universities, please contact the corresponding author.
In Table 2 (Summary Statistics of College Athletics Financial Variables), we see the summary statistics of the three revenue variables drawn from the CAFI database, along with our calculated profit variable, derived from the source data for the 106 universities under review. These four financial variables can be defined in the following manner:
- Donor Contributions: This is the athletic department’s monetary funding coming from groups and individuals above that generated by ticket revenue from all sports in a year by the school.
- Corporate Sponsorships:This is the sum of sponsorships, advertising, licensing, and royalty revenue received by the school’s athletic department in a year.
- Ticket Sales:This is the sum of all revenue from ticket sales to NCAA events for all sports generated by the athletic department at each institution in a year.
- Profit: This is the overall “profitability” of each school’s athletic department operations across all sports. This “bottom-line” figure for each school is derived by subtracting the institution’s total athletic expenses from its total athletic revenue for the year from the Knight Commission on Intercollegiate Athletics’ College Athletics Financial Information (CAFI) database. And note, while all schools ideally aim to operate their athletic programs to have at least some profit (where annual athletic revenue exceeds athletic expenses), some do have negative profit – or operate at losses – when their annual athletic expenses indeed exceed their athletic revenue.
Table 2: Summary Statistics of College Athletics Financial Variables.
N | Mean | Median | Std Dev | Min | Max | |
Donor Contributions | 1286 | 11.0225 | 7.3045 | 12.1805 | – | 204.8768 |
Corporate Sponsorships | 1286 | 3.8194 | 2.3747 | 4.3299 | – | 36.8629 |
Ticket Sales | 1286 | 10.9449 | 8.1410 | 10.7495 | 0.1131 | 57.9658 |
Profit | 1286 | 2.0214 | 0.2995 | 7.4071 | (18.2082) | 156.3727 |
Note: Data for the revenue-related variables come from the CAFI database. They have been adjusted to 2005 dollar values using a BLS (Bureau of Labor Statistics) inflation calculator.
Figure 2 (Annual Averages of the Four College Athletics Financial Variables [Adjusted to 2005 Dollar Values]) shows that even with inflation considered (as the data accounts for inflation by making use of the BLS (Bureau of Labor Statistics) inflation calculator, Corporate Sponsorships and Donor Contributions have both markedly increased over the 2005-2018 time period for college athletic programs. This trend has been driven by the rise in football-related incomes during this time period across Division I programs, especially for the largest programs in the Power 5 conferences. Interestingly, Profit shows an inconsistent pattern, for while the amount of dollars flowing into these programs has grown significantly, so too have the expenses associated with football programs. This is because many universities, especially in recent years, have increased their spending associated with football specifically in an attempt to “keep up” with the most prominent (and in most cases, the largest revenue) programs (18). Finally, the reader should note the inconsistency found in Ticket Sales over the past decade-plus, reflecting the overall challenge for college sports, as with all spectator sports, to generate actual fan attendance at events – including Division I football games (44) – in an era of advancing technology and media availability (25, 46).
Figure 2: Annual Averages of the Four College Athletics Financial Variables (Adjusted to 2005 Dollar Values).
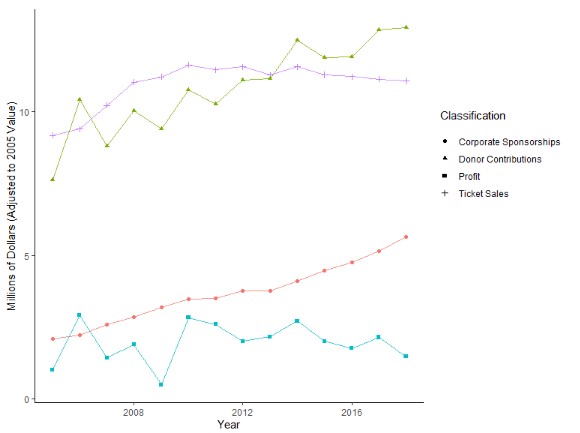
Note: Data comes from the CAFI database and is adjusted to 2005 dollar values by using BLS (Bureau of Labor Statistics) inflation calculator.
Football Data
The source for all football statistical data used in the current study is Sports Reference (4). The data covers the same 2005-2018 time period under review. As stated previously, this research takes into account what are really two different, but definitely related, categories of football performance data. These are first, on-field football team performance, and secondly, the derivative end-of-season data that encompasses both overall team performance and the rewards garnered by the football team for their on-field performance. The latter category includes both measures that are entirely within the team’s control (including the number of wins and their margin of victory) and metrics that are strongly influenced, but not absolutely related, to the team’s overall performance (national poll rankings and bowl game appearances).
In regard to football team performance then, the present research takes into account three categories of performance metrics from the Sports Reference data spanning 2005-2018 to create our football variables. These football variables can be categorized and defined in the following manner:
- Offensive Measures: From Sports Reference’s College Football Team Offense Data:
- Passing TD: This is the per game average number of passing touchdowns scored by the school for the season.
- Rush TD: This is the per game average number of rushing touchdowns scored by the school for the season.
- TO: This is the per game average number of turnovers by the school for the season. It is an indicator of offensive efficiency.
- Defensive Measure: From Sports Reference’s College Football Team Defense Data:
- Opponent TO:This is the per game average number of turnovers by the school’s opponents for the season. It is an indicator of defensive efficiency.
- End-of-Season (Total Team Performance) Measures: From Sports Reference’s College Football Team Data, College Football Standings Data, and College Football Bowl Games Data:
- MOV: This is the average margin of victory (MOV) for the school over the season. This is calculated for all games – both wins and losses – so it is important to recognize that a school could have either a negative or positive margin of victory across the span of an entire season.
- Wins:This is the number of games won by the school in a season.
- Bowl:This is a binary variable indicating whether the school played in a post-season bowl game.
- AP: This is a binary variable indicating whether the school was ranked in the Associated Press’s (AP) year-end Top 25 poll.
Table 3 (Summary Statistics of Football Variables) shows the overall summary statistics in regard to these football variables, with the exception of the two binary variables for bowl game appearances and year-end AP poll rankings.
Table 3: Summary Statistics of Football Variables
N | Mean | Median | Std Dev | Min | Max | |
Passing TDs per Game | 1286 | 1.6540 | 1.6000 | 0.6472 | .3000 | 4.4000 |
Rushing TDs per Game | 1286 | 1.6904 | 1.6000 | 0.6571 | .3000 | 4.2000 |
TOs per Game | 1286 | 1.7043 | 1.7000 | 0.4561 | .4000 | 3.3000 |
Opponent TOs per Game | 1286 | 1.7176 | 1.7000 | 0.4410 | .6000 | 3.4000 |
Margin of Victory | 1286 | 1.4093 | 1.7000 | 11.3505 | (31.1000) | 39.5000 |
Number of Wins | 1286 | 6.6975 | 7.000 | 3.0996 | – | 15.0000 |
School and State Control Data
The present research employs four variables to account for differences between the institutions and to control for that effect as we look at the impact of the football variables specifically. The source for institutional characteristics data utilized in this study is the National Center for Education Statistics (NCES) Integrated Postsecondary Education Data System’s (IPEDS) database (31). The four school-related control variables can thus be defined in the following manner:
- P5:This is a binary variable indicating whether the university is a member of one of the Power 5 Conferences (consisting of the present Power 5, Atlantic Coast Conference, Big Ten Conference, Big 12 Conference, Pac-12 Conference, and Southeastern Conference, and the Big East Conference prior to conference realignment and the end of the BCS [Bowl Championship Series] period in 2014 (14).
- Flagship:This is a binary variable indicating whether the institution is its state’s flagship university (i.e., the most known university in each state).
- Grad Rate: This is each institution’s 6-year graduation rate for a given year.
- Enrollment:This is thetotal enrollment at each institution divided by 1000 for a given year.
The present study also uses state characteristics to account for differences between the locations of institutions across the country. The main source for data on state-specific characteristics is the Federal Reserve Economic Data (FRED) database, published by the Federal Reserve Bank of St. Louis (11). From the FRED database, the researchers garnered four state-specific variables to characterize the economy of the state in which each institution is sited. These four state-related control variables are:
- Population: This is each institution’s state population divided by 1 million in a given year.
- Bachelors:This is the percentage of each institution’s state population that has a bachelor’s degree or higher in a given year.
- GDP:This is the annual per capita GDP (Gross Domestic Product) for the state in which the institution is located, calculated by dividing the state’s GDP divided by state population’s in a given year (expressed in thousands of dollars).
- Unemployment Rate:This is the annual unemployment rate for the state in which the institution is located.
Table 4 (Summary Statistics of School and State Control Variables) presents summary statistics for both the four school and four state control variables employed in the present research.
Table 4: Summary Statistics of School and State Control Variables.
N | Mean | Median | Std Dev | Min | Max | |
School Control Variables: | ||||||
6 Year Graduation Rate | 1286 | 61.8387 | 60.8000 | 15.2253 | 26.9000 | 94.6000 |
Enrollment (000) | 1286 | 29.6937 | 28.1600 | 11.2543 | 8.5170 | 73.3780 |
State Control Variables: | ||||||
State Population (M) | 1286 | 10.0558 | 6.5054 | 9.2673 | 0.5227 | 39.4616 |
Population that Hold Bachelor’s Degree or Higher (%) | 1286 | 27.2067 | 26.6000 | 4.6595 | 16.5000 | 41.7000 |
State GDP per Person (000) | 1286 | 48.2044 | 47.0179 | 8.9809 | 29.5593 | 85.4499 |
State Unemployment Rate (%) | 1286 | 6.3092 | 5.8042 | 2.2716 | 2.4342 | 13.6584 |
Methodology and Hypothesis
The current research makes use of regression analysis to explore the relationship between football performance variables and four variables reflecting the financial performance of the school’s college athletics department overall. Controlling factors in the regressions are considered to be two-fold, encompassing both the characteristics of the institution itself and the economy of the state in which the respective university is located. Through such an econometric analysis of Division I universities, the present study lends insights into how on-field football performance impacts the financial state of universities and their athletic programs overall today.

The hypothesis under review (shown in the econometric equation above, henceforth referred to as Formula 1) looks at the impact of football performance variables on the financial performance variables in the same (the observation) year. The supposition is that passing and rushing touchdowns will have a positive impact on Donor Contributions, Corporate Sponsorships, and Ticket Sales, while turnovers by the offense will have a negative impact on these same three financial metrics. On the defensive side, we believe that opponent turnovers will have a positive relationship with these three financial metrics. The researchers also posit that the cumulative performance variables, both those under the team’s control (margin of victory and wins) and those outside of the team’s control (AP poll ranking and bowl game appearance) will have a positive effect on Donor Contributions, Corporate Sponsorships, and Ticket Sales. Finally, the researchers do not anticipate there being a consistent relationship between any of the football performance variables and Profit. While one would anticipate that having a more successful (i.e. winning) football team would correlate to a more profitable athletic department overall, as discussed previously, the vagaries and variances to be found in each university’s athletic spending across the board will make this relationship less clear and consistent than with the other three financial metrics.
Formula 1 will be used in the regressions on the four college athletics financial performance variables, with the football performance variables noted as follows (and please note that for all variables, the subscripts i and t represent the institution and the year of observation):
- Pass TDi,t is the average number of passing touchdowns per game earned by an institution’s football team in the observation year;
- Rush TDi,t is the average number of rushing touchdowns per game earned by an institution’s football team in the observation year;
- TOi,t is the average number of offensive turnovers committed per game by an institution’s football team in the observation year;
- Opponent TOi,t is the average number of offensive turnovers committed by the opposing team’s offense per game in the observation year;
- MOVi,t is the average number of points by which the institution’s football team wins or loses games. Winsi,t is the number of wins the institution’s football team earns during the observation year;
- Bowli,t is a binary indicator that designates a value of 1 if the institution’s football played in a post-season bowl game; and
- APi,t is a binary indicator that designates a value of 1 if the institution’s football team ended the year in the Associated Press’s year end Top 25 poll.
RESULTS
This section presents the results of the regressions to test the hypothesis under review in the present study and the researchers’ interpretations of the findings from the total of 1286 observations gathered for the 106 Division I during the 2005-1018 time period under review.
Table 5 (Results of Observation Year Regressions on Football Variables) displays the results of our regressions on the four athletics financial performance variables with football performance variables from the observation year (i.e., the same year).
Table 5: Results of Observation Year Regressions on Football Variables.
Donor Contributions ($M) | Corporate Sponsorships ($M) | Ticket Sales ($M) | Profit ($M) | ||||||
Team Performance Variables: | |||||||||
Pass TD | 0.8791 | * | 0.2747 | (0.3668) | (0.1000) | ||||
Rush TD | 1.2557 | * | 0.6295 | *** | 0.4043 | 0.6480 | |||
TO | (1.5484) | ** | (0.0009) | (0.4832) | (0.0890) | ||||
Opponent TO | (1.7838) | ** | (0.8610) | *** | (2.3733) | *** | (0.9220) | ||
MOV | 0.0517 | (0.0007) | 0.0656 | 0.0630 | |||||
Wins | (0.2453) | (0.0195) | 0.0692 | (0.1045) | |||||
Off the Field Team Variables: | |||||||||
Bowl | 0.9507 | 0.1503 | 0.4528 | 0.2675 | |||||
AP | 3.2930 | *** | 0.5738 | 2.9781 | *** | 1.5056 | * | ||
School Control Variables: | |||||||||
P5 | 10.8092 | *** | 1.9526 | *** | 9.6300 | *** | 1.7275 | ** | |
Flagship | 2.5340 | *** | 1.8443 | *** | 5.3019 | *** | 0.9288 | ||
Grad Rate | 0.0991 | *** | 0.0411 | *** | 0.0873 | *** | 0.0258 | * | |
Enrollment | 0.0533 | 0.1068 | *** | 0.1632 | *** | 0.0500 | |||
State Control Variables: | |||||||||
Population | 0.3013 | *** | 0.0710 | * | 0.0511 | 0.0832 | |||
Bachelors | (0.3795) | *** | (0.1821) | *** | 0.7243 | *** | (0.1647) | ** | |
GDP | (0.0054) | 0.0888 | *** | 0.1321 | *** | (0.0324) | |||
Unemployment | (0.0000) | *** | (0.0000) | (0.0000) | (0.0000) | ||||
Constant: | 8.8006 | ** | (3.4111) | *** | 10.1190 | *** | 4.4354 | * | |
N Obs. | 1286 | 1286 | 1286 | 1286 | |||||
R2 | 0.4784 | 0.4851 | 0.7133 | 0.1136 | |||||
Adjusted R2 | 0.4718 | 0.4786 | 0.7097 | 0.1024 | |||||
Discussion
Through the regression analyses, the researchers found that far more of the variability to be found between the 106 Division I college athletics programs’ financial performance could be explained by the control variables – both in regard to the characteristics of the institutions themselves and the demographic and economic attributes of the states in which they are located – than the actual performance of each university’s football team. This is not to be regarded as a surprising finding in any way, as indeed, the nature of the university and the state in which it is sited should be strongly correlated with the overall financial performance of the school’s athletic program. Yet, the absolute strength of these rather constant forces demonstrates just how important it is for university and athletic leaders to recognize the “lay of the land” in their locale and how their unique institutional and location situation impacts – and in many cases, restricts – just how competitive their athletic programs can be – from a financial perspective – in the seemingly ever-increasingly competitive world of college athletics today. The importance of these situational variables does appear to be an important consideration for universities today, and as such, the researchers plan to conduct a follow-up study to specifically investigate the role of both location and institutional factors in this regard.
With that being said however, the regressions do also show that the “football factor” has an impact – and in some cases, it has a quite significant impact – on the financial performance of college athletics departments, specifically the four college athletics financial performance variables (Donor Contributions, Corporate Sponsorships, Ticket Sales, and Profit). In examining the findings in regard to the eight-football performance related variables, the researchers will show how the results of the regressions support the fact that exciting – and winning – football does matter in terms of producing better financial outcomes for college athletic programs overall.
In regard to the “excitement” factor, there were some interesting and revealing findings from our research. First, there is the matter of scoring measures, which in this study are represented by Passing and Rushing Touchdowns. These measures were found to be positively related to both Donor Contributions and to Corporate Sponsorships, but with differing levels of significance. However, there was not a significant correlation found between these two offensive metrics and with Ticket Sales or Profits. Next, there is the matter of turnovers – both those committed by the team themselves and by their opponents. Interestingly, both on-field performance metrics relating to turnovers – both those committed by the team’s offense and achieved by their defense – were found to be negatively correlated with the financial variables under review for the most part, and with some significant relationships to be found, especially in regard to turnovers committed by opponents. This can be taken as a sign that good, exciting football – with high-quality play by both teams (with a lack of turnovers and high scoring being good indicators of such) – is a positive attribute for the quality of play that does enter into the financial equation for institutions.
The results of the regressions also reveal interesting findings in terms of the matter of what winning on the field means in terms of financial metrics for the athletic program overall. First, perhaps not surprisingly when dealing with such a large number of football programs (106) over more than a decade, both the number of wins and the margin of victory statistics “evened out” and were not found to be significant in the regression analysis. While the fact that a team appeared in a bowl game was not found to be significant, whether a team appeared in the final AP rankings was quite significant in terms of the positive impact on financial performance. With the well-documented proliferation of bowl games (43), which has made it both difficult and even controversial for all bowls to find eligible teams to play in them (10), the bowl game finding should not be surprising. However, with the AP ranking being an indicator of not just an institution having a winning program, but one that finds the university as having a program regarded among the nation’s best upon the conclusion of the season, one can interpret the findings as meaning that fans – and monetary supporters today – can delineate the difference between simply having a team that is not simply “bowl eligible,” but one that performs well enough on the field to be ranked in the top 25 in the nation.
In sum, while the correlations found between the eight football performance measures and the four financial metrics of college athletic operations were not as strong as anticipated, especially as compared to the control variables relating to the school and its location, there was a logic and a soundness to the results from the 1286 observations included in the three regressions. The findings demonstrate that in spite of the range of programs included in the study dataset, football programs that produce a higher quality, more exciting brand of football on the field will help generate better financial performance for their institutions overall. The weakest link is again with the profit measure, and this can be explained by the wide variance to be found in the profitability of college athletic programs today due to the vast differences in athletic expenditures today (34). Overall, the present research demonstrates that a quality product on the field does matter in the competitive college sports marketplace – both on and off the field – today.
Conclusions
This study began by placing college football in the context of the overall structure of typical college athletic programs today, wherein the football program is most often the driver of the majority of revenue for the entire athletic operation of the institution. Better understanding the relationship between on-field football performance and the overall athletic department’s financial performance in colleges and universities today is thus not only important for better university and athletic administration. Indeed, this is a critical research gap in the sport and economics literature that the present research has sought to examine for the first time. As such, this study is an initial, but pioneering, work in exploring the relationship between what happens on the college football field and how this impacts the overall health and financial performance of the institution’s athletic program.
The results of the current study do shine a spotlight on relationships to be found between actual football team performance and the financial performance of the athletic program of the institution overall. As we have seen, the findings of the current research highlight the fact that there are a variety of connections to be found between specific aspects of football team performance and the four college athletic department measures surveyed in this study. These were detailed in the preceding section, with the present analysis having demonstrated that exciting, winning football does play an explanatory role in the financial performance of athletic department operations overall.
Looking ahead, the researchers look forward to seeing additional research being done in both the sport and economics fields to investigate the relationship between the on-field performance of college football teams and the financial performance of college athletics departments. One could see future contributions coming not just in the form of further econometric studies, but perhaps using other research approaches and arising in the fields of higher education studies and public administration as well. However, given the type of data that needs to be incorporated into such studies – football performance metrics and financial outcomes measures, it would appear that econometric analyses are the most appropriate way to approach studying the relationships between football and overall athletic program financial performance.
The current study represents a significant contribution to the literature on the economics of sports and the line of research that has examined sports from an econometric perspective. It stands as an initial attempt that links on-field college football performance with the financial performance of college athletic departments for institutions, and hopefully, subsequent research will build upon the present research to help better understand this critical equation for college athletics now and into the future.
Applications in Sport
With the importance of football in the economics of college sports today for every institution in America, better understanding the connection between football performance and the overall financial performance of the athletic department is vital for all stakeholders not just in college athletics, but in universities in general. As a diversified enterprise, college athletics programs and their administrators benefit from research such as that conducted in the present research, and the researchers look forward to much more activity in this same vein in the future to further explore the connection between on-field football performance and the financial success – and challenges – for college athletics programs overall.
For athletic departments and athletic administrators, the findings in the present research provide them with new insights on what donors, sponsors, and yes, fans of their football programs value in terms of on-field performance of their respective university’s football team. Yes, football’s bottom-line measures – wins and ultimately national rankings – do matter to their constituencies. While athletic programs, and certainly football coaches and their staff, may tout bowl game successes for their football teams, with the proliferation of bowl games, this may be of far lesser regard today in the minds of donors, sponsors, and ticket-buyers than athletic and even university administrators presently believe.
In regard to what kinds of football appeal to fans, this study shows that excitement factors – with high levels of offense and good, quality play – may mean more to the athletic department’s bottom-line (in terms of revenue, donations, and sponsorships) than even wins and losses. While competitive pressures can and should dictate football coaches decision-making, one cannot help but recognize that today, in light of our findings, head coaches and their staff members might just need to rethink the true value of winning games in “unexciting ways” (e.g. with scores like 12-10 or 9-0). Winning in an unexciting fashion may thus not be nearly as valuable – from a bottom-line financial perspective – to the university’s athletic department and its budget as when there is more excitement generated on the field. So too might winning against an unappealing schedule, as when Power 5 schools play too many weak opponents, often from the FCS ranks, today during a season. As such, our study pinpoints that yes, in college football today, winning, in and of itself, may not be enough – at least from a financial standpoint!
Acknowledgements
The authors disclose no conflicts of interest or funding may influence these data or the interpretation thereof. There were no funding sources for this research.
References
- Arkes, J. (2011). “Is controlling the rushing or passing game the key to NFL victories?” The Sport Journal, 14(1), 2011. Retrieved from https://thesportjournal.org/article/is-controlling-the-rushing-or-passing-game-the-key-to-nfl-victories/.
- Baumann R., & Matheson V.A. (2013). Estimating economic impact using ex post econometric analysis: cautionary tales. In Rodriguez P., Kesenne S., Garcia J. (eds), The Econometrics of Sport (pp. 169-188). Cheltenham, UK: Edward Elgar Publishing.
- Bernard, A.B., & Busse, M.R. (2004). Who wins the Olympic Games: Economic resources and medal totals. The Review of Economics and Statistics, 86(1): 413-417.
- Bigalke, Z. (2019, June 23). Will more schools take Idaho path from college football’s FBS to FCS? Saturday Blitz, Retrieved from https://saturdayblitz.com/2019/06/23/college-football-more-schools-take-idaho-path-fbs-fcs/z.com/2019/06/23/college-football-more-schools-take-idaho-path-fbs-fcs/.
- Boston Consulting Group (n.d.). What is the Growth Share Matrix? Retrieved from https://www.bcg.com/en-us/about/our-history/growth-share-matrix.
- Bowen, F. (2020, October 28). This strange college football season could have lasting effects: Fans and traditional rivalries will return, but cuts to athletic programs may be permanent. The Washington Post, Retrieved from https://www.washingtonpost.com/lifestyle/kidspost/this-strange-college-football-season-could-have-lasting-effects/2020/10/28/a5a8cc90-0819-11eb-a166-dc429b380d10_story.html.
- Buts, C., Bois, C. D., Heyndels, B., & Jegers, M. (2013). Socioeconomic determinants of success at the Summer Paralympics Games. Journal of Sports Economics, 14(2), 133–147.
- Cannon, J. (2020, October 11). Seasons derailed, programs cut: Athletes face emotional blow as pandemic uproots college sports. USA Today, Retrieved from https://www.usatoday.com/story/news/education/2020/10/11/college-sports-cut-seasons-canceled-student-athletes-feel-abandoned/3585109001/.
- Del Corral, J., Barros, C. P., & Prieto-Rodríguez, J. (2008). The determinants of soccer player substitutions: A survival analysis of the Spanish Soccer League. Journal of Sports Economics, 9(2), 160–172.
- Dodd, D. (2019, November 26). College football hoping to avoid its first shortage of bowl-eligible teams since 2015: With more bowl games to be added, questions remain about the quality of the bowl system. CBS Sports, Retrieved from https://www.cbssports.com/college-football/news/college-football-hoping-to-avoid-its-first-shortage-of-bowl-eligible-teams-since-2015/.
- Federal Reserve Bank of St. Louis (2020). Federal Reserve Economic Data (FRED) Database. Retrieved from https://fred.stlouisfed.org.
- Forrest, D., Sanz, I., & Tena, J.D. (2010). Forecasting national team medal totals at the Summer Olympic Games. International Journal of Forecasting, 26(3), 576‒ 88.
- Fullerton, S.L, Fullerton, T.M., Jr., & Walke, A.G., (2014): An econometric analysis of the 2013 Major League Baseball season. Research in Business & Economics Journal , 9(4): 1-6.
- Gall, B. (2013). History of Big East realignment; Birth of the American Athletic Conference. Athlon Sports, Retrieved from https://athlonsports.com/college-football/history-big-east-realignment-birth-american-athletic-conference.
- Garthwaite, C., & Notowidigdo, M. (2020, October 16). The COVID-19 pandemic is revealing the regressive business model of college sports. The Brookings Institution. Retrieved from https://www.brookings.edu/blog/brown-center-chalkboard/2020/10/16/the-covid-19-pandemic-is-revealing-the-regressive-business-model-of-college-sports/.
- Giratikanon, T., et. al. (2014, October 3). N.C.A.A. fan map: How the country roots for college football – With data based on Facebook ‘likes’, we estimated the boundaries of fandom for 84 teams. The New York Times, Retrieved from https://www.nytimes.com/interactive/2014/10/03/upshot/ncaa-football-fan-map.html.
- Gladwell, M. (2019, November 5). More on quarterbacks. Gladwell.com, Retrieved from https://gladwell.typepad.com/gladwellcom/2009/11/more-on-quarterbacks.html.
- Glier, R. (2020, August 13). College football programs, spending money as fast as they make it, have little In reserve for an endangered season. Forbes, Retrieved from https://www.forbes.com/sites/rayglier/2020/08/11/hey-college-football-caretakers-where-is-37-billion/?sh=463bbdff7fc6.
- Haas, D., Kocher, M.G., & Sutter, M. (2004). Measuring efficiency of German football teams by data envelopment analysis. Central European Journal of Operations Research, 12(3): 251-268.
- Henderson, B. (1970). Boston Consulting Group: The Product Portfolio. Retrieved from https://www.bcg.com/en-us/publications/1970/strategy-the-product-portfolio.
- Jianakoplos, N. A., & Shields, M. (2012). Practice or profits: Does the NFL preseason matter? Journal of Sports Economics, 13(4), 451–465.
- Katz, S. & Burke, B. (2016, September 8). How is Total QBR calculated? We explain our quarterback rating. ESPN.com, Retrieved from https://www.espn.com/blog/statsinfo/post/_/id/123701/how-is-total-qbr-calculated-we-explain-our-quarterback-rating.
- Knight Commission on Intercollegiate Athletics (2020). College Athletics Financial Information (CAFI) Database. Retrieved from http://cafidatabase.knightcommission.org.
- Lee, Y.H., & Berri, D. (2008). A re‐examination of production functions and efficiency estimates for the national basketball association. Scottish Journal of Political Economy, 55(1); 51-66.
- Leitch, W. (2018, July 11). Nobody’s going to sports in person anymore. And no one seems to care. New York Magazine, Retrieved from https://nymag.com/intelligencer/2018/07/nobodys-going-to-sports-in-person-and-no-one-seems-to-care.html.
- Loumena, D. (2013, October 05). Reggie Jackson tries to set record straight on Thurman Munson quote. The Los Angeles Times, Retrieved from https://www.latimes.com/sports/sportsnow/la-sp-sn-reggie-jackson-autobiography-20131004-story.html.
- Lui, H-K. & Suen, W. (2008). Men, money and medals: An econometric analysis of the Olympic Games. Pacific Economic Review, 13(1): 1–16.
- NCAA (National Collegiate Athletic Association) (2020a). Student-athletes. Retrieved from http://www.ncaa.org/student-athletes.
- NCAA (National Collegiate Athletic Association) (2020b). FBS football: Individual statistics. NCAA.com, Retrieved from https://www.ncaa.com/stats/football/fbs/current/individual/8.
- NCAA (National Collegiate Athletic Association) (2020c). FBS football: Team statistics. NCAA.com, Retrieved from https://www.ncaa.com/stats/football/fbs/current/team/699.
- National Center for Education Statistics (NCES), U.S. Department of Education (2020). Integrated Postsecondary Education Data System. Retrieved from https://nces.ed.gov/ipeds/use-the-data.
- Pestana-Barros, C., Assaf, A., & Sá-Earp, F. (2010). Brazilian Football League technical efficiency: A Simar and Wilson approach. Journal of Sports Economics, 11(6), 641–651.
- Pope, D. G., & Pope, J.C. (2014). Understanding college application decisions: Why college sports success matters. Journal of Sports Economics, 15(2), 107–131.
- Redd, D. (2018, July 16). College athletics arms race shows no signs of slowing. Athletic Business, Retrieved from https://www.athleticbusiness.com/college/college-athletics-arms-race-shows-no-signs-of-slowing.html.
- Reilly, B. & Witt, R. (2013) Red cards, referee home bias and social pressure: evidence from English Premiership Soccer, Applied Economics Letters, 20(7): 710-714.
- Scoppa, V. (2008). Are subjective evaluations biased by social factors or connections? An econometric analysis of soccer referee decisions. Empirical Economics, 35(1): 123–140.
- Sherman, R. (2020, November 6). The game that defined the weirdest season in college football history. The Ringer, Retrieved from https://www.theringer.com/2020/11/9/21557054/notre-dame-clemson-game-that-defined-the-season.
- Shibli, S., Gratton, C., & Bingham, G. (2012) A forecast of the performance of Great Britain and Northern Ireland in the London 2012 Olympic Games, Managing Leisure, 17(2), 274-290.
- Smith, C. (2019). College football’s most valuable teams: Reigning champion Clemson Tigers claw into top 25. Forbes, September 12, 2019. Retrieved from https://www.forbes.com/sites/chrissmith/2019/09/12/college-football-most-valuable-clemson-texas-am/#cbcea77a2e7e.
- Sports Reference (2020). College Football Statistics. Retrieved from https://www.sports-reference.com/cfb/.
- Stewart, M, Mitchell, H & Stavros, C (2007), Moneyball applied: Econometrics and the identification and recruitment of elite Australian footballers. International Journal of Sport Finance, vol. 2, no. 4, pp. 231-248.
- Van, C. (2020, October 16). Pay to play in the NCAA: A data driven playbook on how to compensate athletes, The Sport Journal, 21(1). Retrieved from https://thesportjournal.org/article/pay-to-play-in-the-ncaa-a-data-driven-playbook-on-how-to-compensate-athletes/.
- Whitney, C. (2020, January 15). Too many college football bowl games, too few fans. Gaming Today, Retrieved from https://www.gamingtoday.com/race_sports/ncaa/article/88333-Too_many_college_football_bowl_games_too_few_fans.
- Wilder, C. (2020, January 10). Unexcused absence: Why is college football attendance tanking? Sports Illustrated, Retrieved from https://www.si.com/college/2020/01/10/college-football-attendance-decline-ncaa.
- Wyld, D.C. (2019, September). College football, inc.: The Saturday tradition has become a very, very big — and profitable — business today. Is that all bad?, The Startup, Retrieved from https://medium.com/swlh/college-football-inc-284a152a521e.
- Wyld, D. (2018, July 18). The now constant crisis of empty seats in sports. Unbalanced, Retrieved from https://vocal.media/unbalanced/the-now-constant-crisis-of-empty-seats-in-sports.